Our first real contact with the JL user base was through an introductory survey. We wanted to better understand how people use the file browser as it is, what they disliked about it, and what they wanted to see next. We quickly discovered that the vast majority of those surveyed were developers or data scientists who considered themselves of mid- to expert-level proficiency.

We interviewed 10 JupyterLab users of varying expertise levels and occupations. They ranged from key stakeholders to students, and revealed three feature-level challenges:
Lack of functionality: Feature does not exist.
Lack of discoverability: Feature exists but users do not know it does.
Lack of feedback: Feature exists but users do not trust it.
From these interviews, the key quotes, pain points, and touch points would be used to craft personas and journey maps, and we were also able to extract a rudimentary feature request chart.
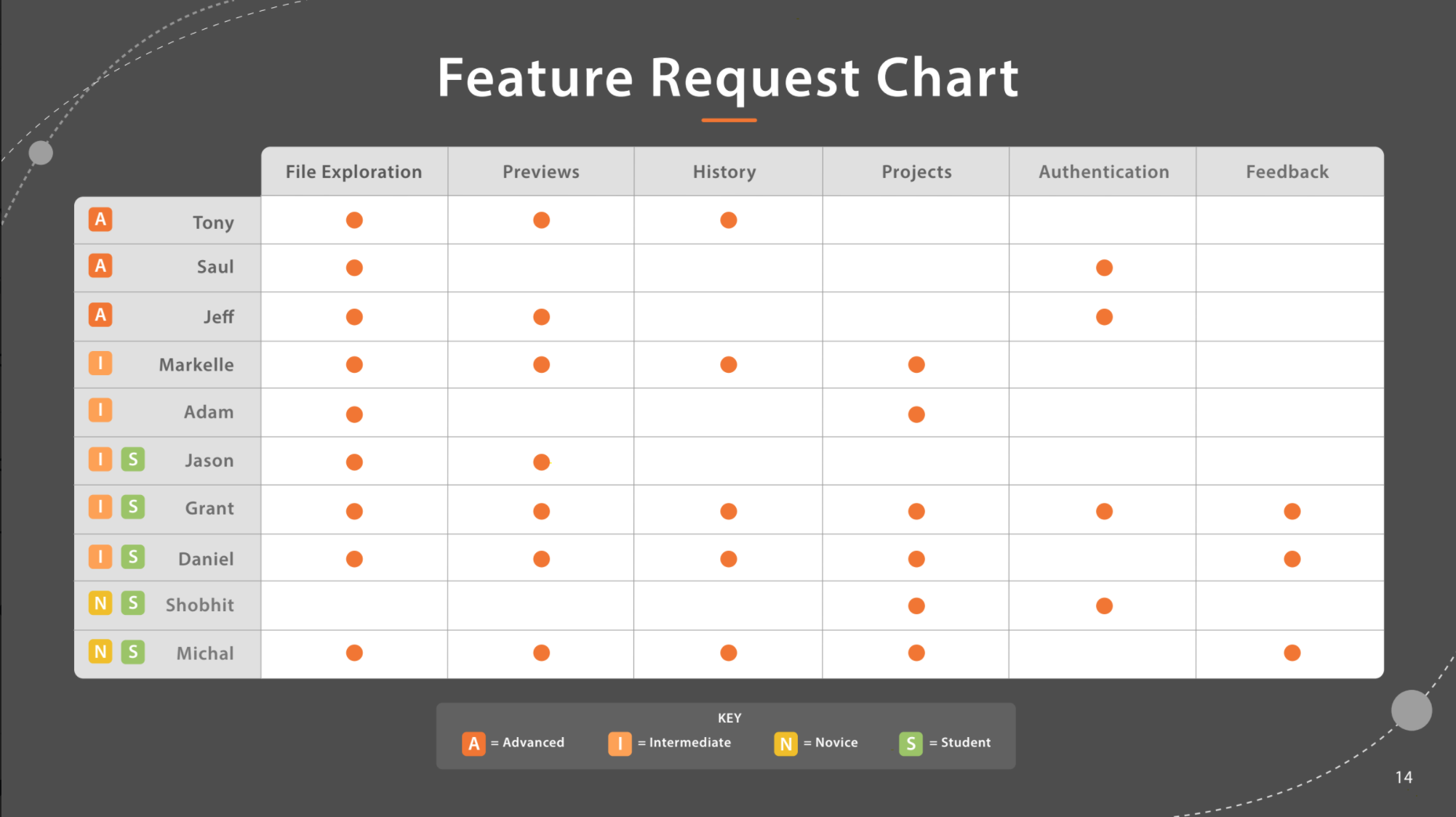
Personas and Journey Mapping
Our research until this point in the project had revealed that JupyterLab’s user base is diverse in both occupation and expertise. We identified 4 key personas who made up the vast majority of users: the data scientist (expert), the developer (expert), the intern (intermediate), and the student (novice).
Each user’s journey was mapped from spinning JupyterLab up, till the end of their work flow. While the journeys were highly variable, every persona had 2 common pain points: finding files, and organizing them once found. We would center our design prompt and requirements around these two points once we began the problem solving phase of the project.
